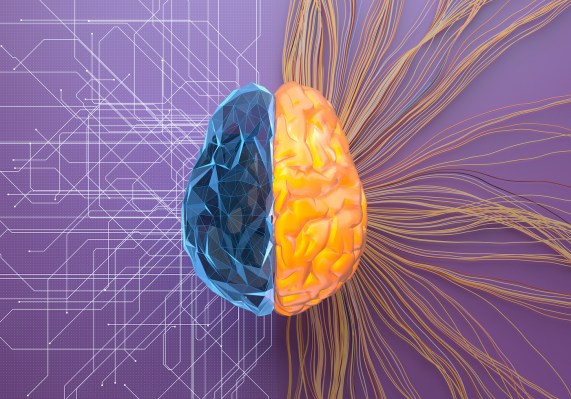
Founding founders often believe that responsible AI practices can be difficult to implement and could slow down their business' progress. Many founders look to established examples such as Salesforce's Office of Ethical and Humane Use and believe that building a large team is the only way to avoid creating harmful products. It is actually much simpler.
I spoke with several early-stage founders who were successful in implementing responsible AI practices.
They didn't call it that. They call it good business.
Simple practices that are business-friendly and lead to better products can make a big difference in reducing the likelihood of unintended societal harms. These practices are based on the understanding that humans, not data, are the key to successfully deploying an AI solution. You can create a more responsible business if you consider the fact that humans are always in control.
AI can be described as a bureaucracy. AI is a bureaucracy that relies on a general policy (the model) to make reasonable decisions in most cases. This policy cannot account for all possible situations that a bureaucracy may encounter. An AI model, however, cannot anticipate every possible input.
These policies or models can fail to reach the people who are already marginalized. A classic example of this is Somali immigrants being tagged as fraud for their unusual shopping habits.
This problem is solved by bureaucracies. They have street-level bureaucrats such as judges, DMV agents, and teachers who can handle individual cases or decide to not enforce the policy. Teachers can waive course prerequisites in certain circumstances. Judges can also be more or lesslenient in their sentencing.
Artificial intelligence will eventually fail. We must, like a bureaucracy, keep humans informed and design with them. One founder said to me that if I was a Martian arriving on Earth, I would think: Humans processing machines. I should use them.
These people decide how an AI-based solution in the real world will perform, whether they are the operators who augment the AI system when it is uncertain or the users who choose to accept, reject or manipulate a model outcome.
These are five tips that founders and executives of AI companies have shared to keep humans informed about AI, harnessing it, and building a more responsible AI that is also profitable:
Only use as much AI as you are able to.
Many companies are planning to launch services that use an AI-driven end-to-end process. People who are already marginalized often suffer the most when these processes fail to work under wide-ranging use cases.
When trying to diagnose failures founders take out one component at a while, but still hope to automate as much of the process as possible. They should also consider the reverse: only introducing one AI component at once.
Even with all the AI marvels, many processes are still cheaper and more reliable when run with humans. It can be difficult to determine which components are most suitable for AI if you have many components in an end-to–end system.
Many founders we spoke with view AI as a way to delegate the most time-consuming, low-stakes tasks in their system away from humans, and they started with all human-run systems to identify what these important-to-automate tasks were.
The second AI approach allows founders to venture into areas where data is not readily available. People who manage parts of a system create the data necessary to automate these tasks. We were told by one founder that without his advice to slowly introduce AI and only when it was more accurate than an operator, the company would not have been able to get off the ground.
Induce friction
Many founders believe that a product should be able to run straight out of the box with minimal user input in order to succeed.
A lot of AI is used to automate a part of an existing workflow. This can lead to a catastrophic approach.
An ACLU audit revealed that Amazon's facial recognition tool misidentified 28 members of Congress as criminals. This was due to lax default settings. If a user accepts a positive result as true, the accuracy threshold was set at only 80%.
It is possible to reduce the risk of mismatches by encouraging users to be more engaged with the strengths and weaknesses of a product before they deploy it. Customers will be happier with the product's performance.
According to one founder, customers used their product more efficiently if they had to personalize it before using. This is a key component of a design first approach. He believes it helps users to use the product's strengths on a context-specific level. This approach took more time upfront, but it resulted in revenue increases for customers.
Don't just give answers, but context
A lot of AI-based solutions are focused on the output recommendation. These recommendations must be implemented by humans once they are made.
Poor recommendations can be blindly followed without context, which could lead to downstream harm. Great recommendations can also be rejected if there is no context and trust in the system.
Instead of delegating decision-making to users, give them the tools they need. This approach uses the power of humans to identify problems in model outputs and secures user buy-in for a product that is successful.
One founder said that users distrusted AI's direct recommendations. Customers were pleased with the accuracy of their predictions, but users ignored them. They decided to scrap the recommendation feature and instead use their model to increase the resources that could help users make a decision (e.g. this procedure is similar to these five previous procedures and here's what worked). This resulted in increased revenue and adoption.
Think about your not-users as well as not-buyers
Enterprise tech products often serve the CEO, not end users. This is a problem. This problem is magnified in the AI space where solutions are often part of a larger system that interfaces only with a few users directly.
Consider, for instance, the controversy surrounding Starbucks' use of automated scheduling software to assign shifts. The scheduling software was optimized for efficiency and completely ignored working conditions. The baristas' input was considered after a successful labor petition.
Instead of focusing on the customer's problem and assuming that you can solve it, map out all stakeholders and understand their needs before you determine what your AI will do to optimize. This will help you avoid accidentally creating a product that is harmful or even worse, and may also allow you to find a better business opportunity.
One founder, whom we spoke to, took this approach seriously and walked alongside their customers to learn their needs before deciding on the best product. To make sure that their product was a success for customers and union members, they met with them.
Customers initially wanted a product that allowed each user to handle a larger workload. However, these conversations revealed a way to save money for customers by optimizing their existing workload.
This insight enabled the founder to create a product that empowers the people in the loop and saves management money.
Make sure you understand what AI theater is
You can avoid irresponsible consequences by limiting the hype surrounding what your AI can do and help to sell your product better.
Yes, AI hype can help sell products. It is important to know how to avoid using buzzwords that get in the way precision. Although it might be a good idea to talk about the autonomous capabilities of your product, it could backfire if you use that rhetoric in an indiscriminate manner.
One founder we spoke with said that promoting their AI power also raised privacy concerns for their customers. Even though the founders clarified that the product did not depend on data but on human judgement, this concern persisted.
Language choice can help to align expectations and build trust. Some founders found that using words such as augment and assist with users was more effective than using the language autonomy. AI as a tool framing was less likely to inspire blind trust, which can lead to disastrous outcomes. Clear communication can help you sell and dissuade AI overconfidence.
These are the practical lessons that real founders have learned to mitigate the potential harms of AI and create more long-term products. New startups have the opportunity to develop services that make ethical AI more accessible and also help businesses. Here are some requests from startups:
Involve humans in the loop: Startups that solve the human-in-the-loop attention problem are needed. To delegate to humans, it is important that humans are able to notice when AI is in doubt so they can intervene. Research shows that even if an AI is accurate 95% of time, people tend to get complacent and miss the 5% of errors. We believe that startups in this area can and should emerge from social insights.
Startups that can consolidate existing standards and measure compliance with responsible AI are in a position to establish a standard for responsible AI compliance. As public pressure for AI regulation has increased, the number of AI standards being published has been rising over the past two decades. Recent surveys showed that 84% of Americans believe AI should be managed carefully and rate it as a top priority. This will help companies show that they take this seriously. The current draft of EU's extensive AI Act (AIA), emphasizes industry standards. Compliance will be a requirement if the AIA is passed. We think this market is worth watching, given the existing market for GDPR compliance.
These simple and responsible AI practices will allow you to unlock vast business opportunities, no matter if you're trying them or not. You must be careful when deploying AI to avoid creating harmful products.
This thoughtfulness can pay off in the long-term success and growth of your company.