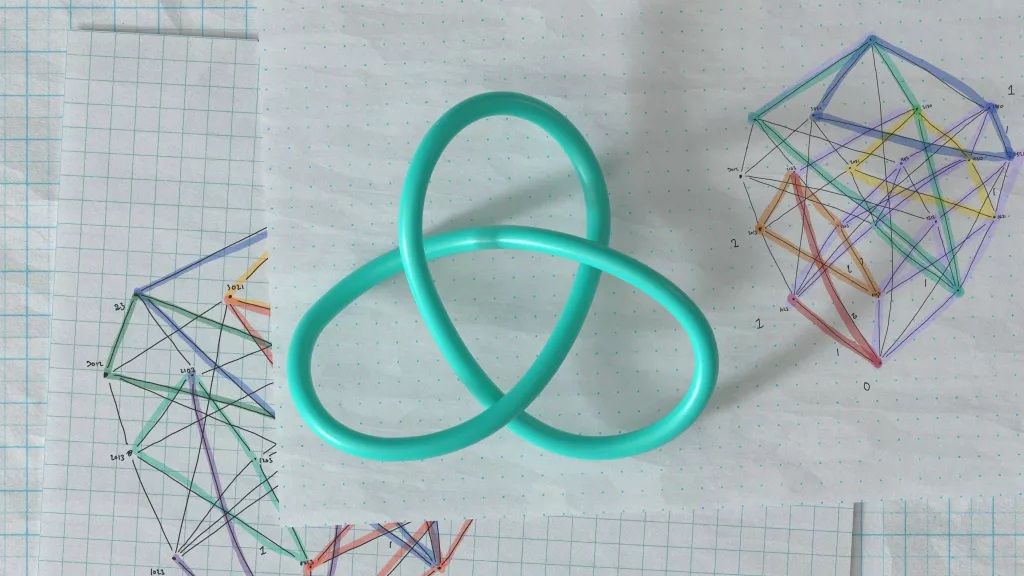
DeepMind, an artificial intelligence program, has revealed a new math problem that may help mathematicians understand knots.
The two pure math conjectures are the first-ever important advances in pure mathematics, generated by artificial intelligence, and were reported in the journal Nature. There are mathematical ideas that are thought to be true but have not been proven in all circumstances. Machine-learning algorithms have been used to generate theoretical ideas in mathematics before, but they have tackled problems smaller than those DeepMind has cracked.
Alex Davies is a machine-learning specialist at DeepMind and one of the authors of the new paper.
Artificial intelligence is learning how to dodge space junk.
Machine learning and math.
A lot of pure mathematics is looking at patterns in numbers and trying to prove that they are real relationships. This can get very complicated when working with multiple dimensions.
Davies told Live Science that machine learning is good at spotting patterns.
Setting DeepMind onto a path was the first challenge. Davies and his colleagues at DeepMind worked with mathematicians from the University of Oxford and the University of Australia to find out what problems artificial intelligence might be able to solve.
knot theory is the mathematical study of knots, and representation theory is a field that focuses on abstract structures such as rings and lattices, and relates those abstract structures to linear equations.
There are a lot of problems.
In understanding knots, mathematicians rely on invariants, which are the same quantities. In this case, they looked at invariants that were the same in equivalent knots, and they found that they were equivalent if you can distort one into another without breaking the knot. Geometric invariants are the measurement of a knot's overall shape, whereas algebraic invariants describe how the knots twist.
Davies said that there was no proven connection between the two things. The researchers decided to use DeepMind to find out if there was a relationship between the two.
They were able to identify a new geometric measurement with the help of the program. This measurement was related to a signature that describes certain surfaces on knots.
The researchers wrote in Nature that the new hypothesis will open up new theorizing in the mathematics of knots.
In the second case, DeepMind helped reveal why the conjecture works by taking a conjecture from the late 1970s.
For 40 years, mathematicians have believed that it is possible to look at a very complex, multidimensional graph and figure out a particular equation to represent it. They haven't figured out how to do it. The mathematicians who first proposed these equations have been linked to the features of the graphs by DeepMind.
Davies said that they were able to train some machine-learning models that were able to predict what the polynomial would be. The team analyzed what features of the graph DeepMind was using to make those predictions, which helped them get closer to a general rule about how the two map to each other. DeepMind has made a lot of progress on the problem.
There are no immediate practical applications for these pure math conjectures, but the mathematicians plan to uncover more relationships in these fields. The research team hopes that their success will encourage other mathematicians to use artificial intelligence.
Davies wants to encourage people to use this technique and go out there and find new and exciting things.
Live Science published the original article. Follow us on social media.