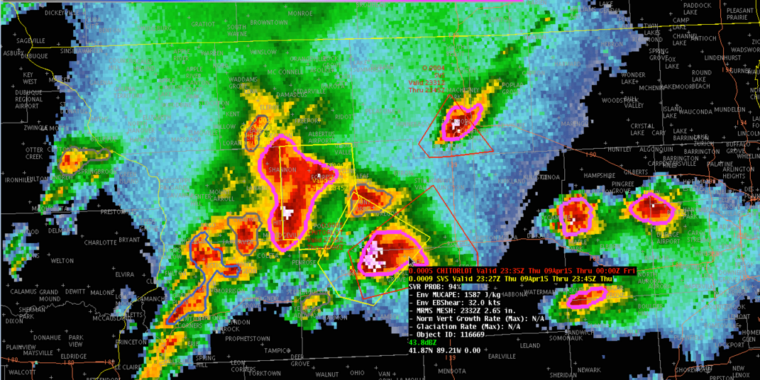
A neural network can infer what radar maps would look like if they were available.
It has become clear that quantum computers won't have a single moment when they are superior to classical hardware. We're likely to see them becoming useful for a narrow set of problems and then gradually expand out from there to an increasing range of computations. One of the questions is where the utility will be seen first.
A case when quantum hardware should offer an advantage has been identified in a white paper by the quantum-computing startup Rigetti. Replacing a neural network that's used for analyzing weather data is useful.
How is the weather?
The people at Rigetti looked at a problem that involved taking a partial set of weather data and inferring what the rest looks like. We only get partial information about local conditions in areas that lack good coverage. If we have things like commercial aircraft going through those areas, we want a complete picture of the conditions there.
Neural networks have been trained to handle this. The system could infer what the rest was likely to be after training. Satellite cloud images and data on lightning strikes can be used by the trained system to create a likely weather radar map.
Neural networks do a good job of recognizing patterns and inferring correlations.
Neural networks also map onto quantum processors, which drew the attention of the Rigetti team. A layer of "neurons" performs operations before forwarding its results to the next layer in a typical neural network. The network learns by changing the strength of the connections. Each qubit can perform the equivalent of an operation on a quantum processor. The strength of the connection can be adjusted by the qubits. Neural networks can be trained on a quantum processor.
Advertisement
It could be better.
The metric that was worked out by the researchers at Google allows for the comparison of artificial intelligence on classical and quantum hardware. The ability to do the comparison is due to the fact that Rigetti has a 32-qubit quantum processor. There are some cases when a quantum system should be better than a classical one.
Exactly what those cases are is not clear. The researchers used their quantum processor as part of a mixed quantum/classical system. The system was more or less successful for certain aspects of the weather data. When reconstructing lightning data using the quantum processor, they found it did a better job at lower altitudes than the classical neural network.
They replaced the neural network with qubits. The quantum version was better for lightning data. When it was tested against satellite data, the tables were turned.
It's important to point out that the quantum system didn't show an actual performance advantage over the existing methods of running this sort of weather analysis, but the indication that better performance is possible. The researchers note that the results show that data in real-world machine-learning problems can have a structure compatible with quantum advantage.
Their ability to perform parts of the analysis on quantum hardware with decent results shows that there's not a barrier to integrating quantum methods into this sort of analysis. This isn't the sort of breakthrough that will grab attention, but it is the sort of hard work that will be needed to get quantum computing to live up to its potential.