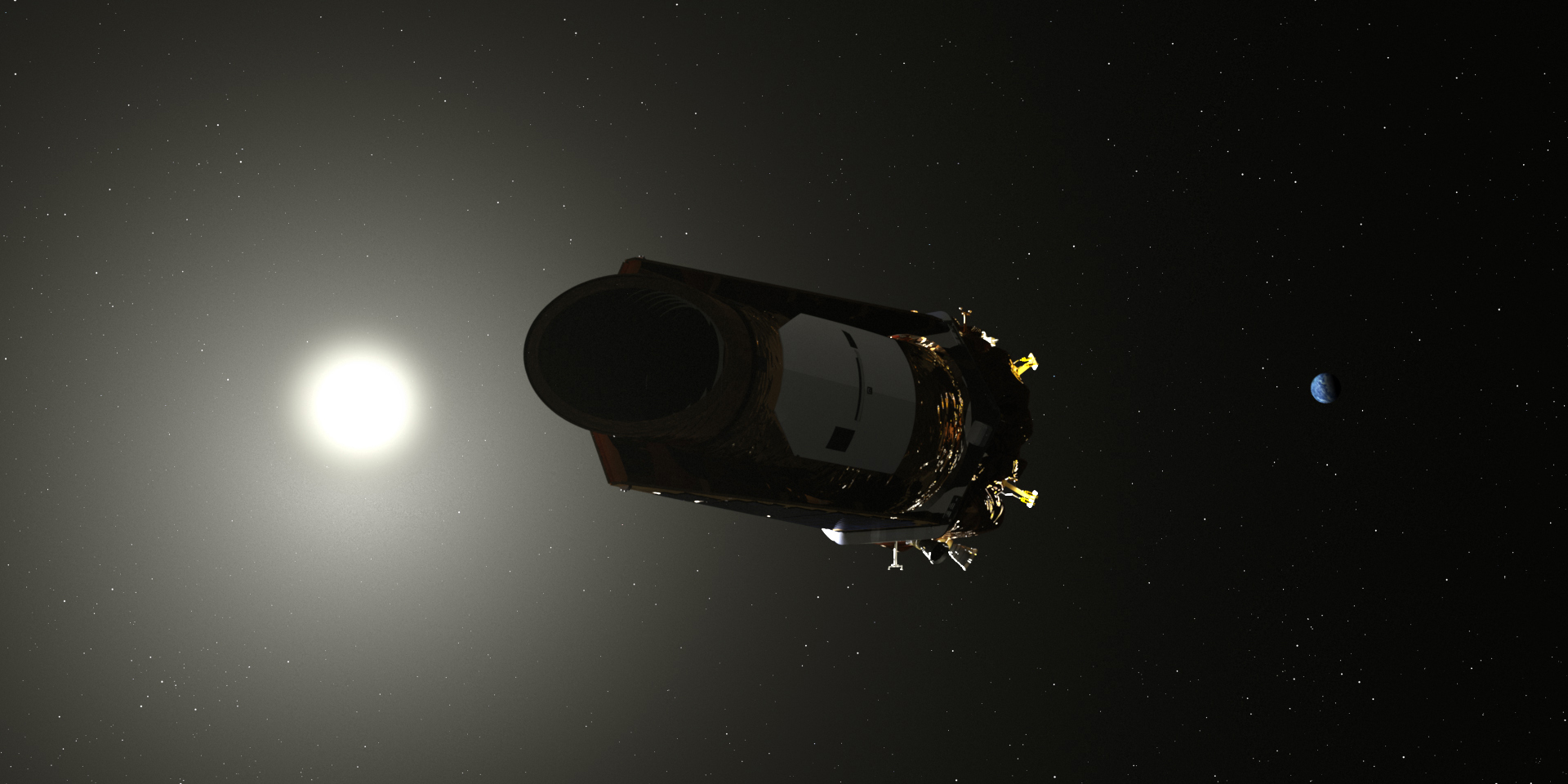
Astronomers are excited to see how machine learning will improve surveys. One field that is benefiting from the search for extrasolar planets is machine-learning, where researchers rely on the technology to distinguish between faint signals and background noise. Machine intelligence is likely to become even more critical as this field transitions from discovery to characterization.
Out of the 4,575 exoplanets discovered made to date, 2879 were accounted for by the Kepler Space Telescope. A research team at NASA's Ames Research Center was able to detect 301 more planetary signals after looking at the data collected by Kepler.
The paper describing these exoplanets and the ExoMiner was accepted for publication in the Astrophysical Journal. The paper and project team were led by Hamed Valizadegan, a machine learning manager with the Universities Space Research Association (USRA) at NASA Ames', and included multiple researchers from the USRA, the SETI Institute, and universities from all around the world.
In their paper, they say that all 301 of the machine-validated planets were originally detected by the Kepler Science Operations Center. The status of these planets was promoted by the science office. No one was able to verify that the potential signals were exoplanets before the analysis of the archive using ExoMiner.
This new deep neural network learns to identify patterns based on the data it has been provided. The researchers at NASA Ames designed the ExoMiner using various tests and properties that human experts use to confirm the presence of exoplanets. This knowledge is combined with NASA's Supercomputer to distinguish between false positives and actual exoplanets.
In the paper, it's indicated that ExoMiner is more precise and consistent in ruling out false positives and identifying signatures of planets while also showing science teams how it arrived at its conclusion. Valizadegan explained.
You can be certain that something is a planet when it says so. Because of the biases that come with human labeling, ExoMiner is meant to be more reliable than both existing machine and human experts. We can transfer the learning from training ExoMiner to other missions with a little tweaking. There is room to grow.
Astronomers use the light curve to determine the presence of planets when a planet crosses between us and a star. The NASA's Goddard Space Flight Center.
Experts who searched through the data gathered during the K2 campaign were assisted by the ExoMiner. The reason for this is that the method used by TESS and the previous one, the Kepler, are related. This consists of monitoring thousands of stars for signs of periodic dips in luminosity, which could be caused by exoplanets passing in front of them.
The transit method is known. Over 75% of all discoveries made to date have been made using transit photometry. It is subject to a high false positives rate, which can be as high as 40% in single-planet systems. It is only effective for 10% of star systems since they must be edge-on relative to the observer for transits to be visible.
The primary way to get around this is to monitor thousands of stars in a single field. Having an automated helpers that can process the data reliably is a huge game-changer. A recent NASA press release states that Jon Jenkins is an exoplanet scientist at NASA's Ames Research Center.
There is no mystery as to why the program decides something is a planet or not. We can easily explain which features in the data lead to rejection or confirmation of a planet.
The newly confirmed planets are not thought to be Earth-like because they are not rocky in composition or in the HZ. The 301 planets are similar to the overall population of confirmed exoplanets in our neighborhood, making them a good addition to the exoplanet census.
Machine learning techniques will prove useful to missions in the future. The TESS is scheduled to remain in operation until Sept. 2022, but also thePLAnetary Transits and Oscillations of stars mission and NASA's Nancy Grace Roman Space Telescope.
Further reading: NASA.