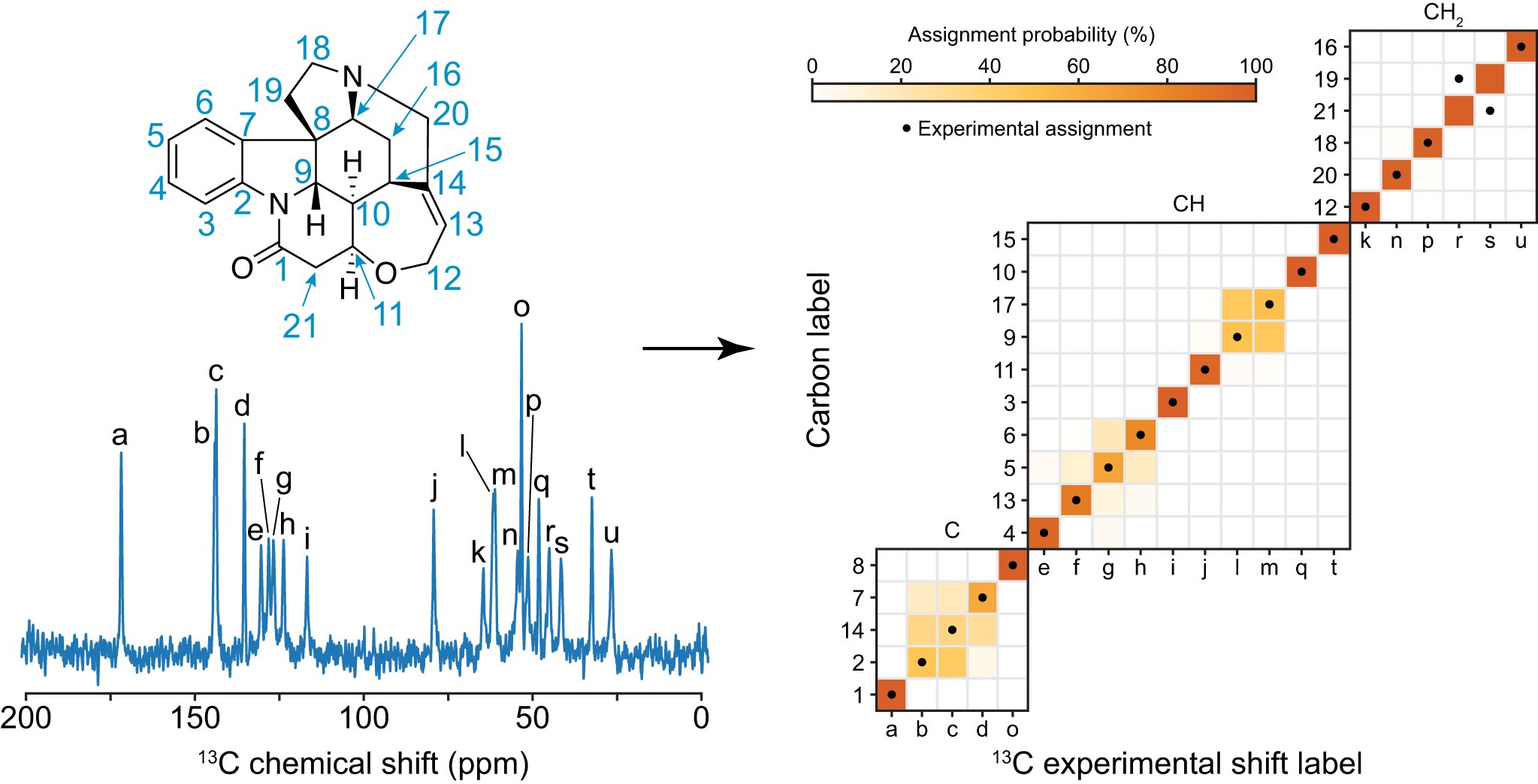
The 13C NMR spectrum of strychnine has a probabilistic assignment. Credit: EPFL.
Solid-state nuclear magnetic resonance is a technique that can be used to determine chemical and 3D structures as well as the dynamics of molecule and materials.
The chemical shift assignment is the first step in the analysis. This involves assigning each peak in the spectrum to an atom. This can be very difficult. Multi-dimensional correlation experiments are usually required to assign chemical shifts. There is no such database for the study of chemical shift.
A team of researchers including EPFL professors Lyndon Emsley, head of the Laboratory of Magnetic Resonance, and Michele Ceriotti, head of the Laboratory of Computational Science and Modeling, decided to tackle this problem by developing a method of assigning NMR spectrum of organic crystals.
They created their own database of chemical shifts by combining the Cambridge Structural Database (CSD), a database of more than 200,000 three-dimensional organic structures, with ShiftML, a machine learning algorithm they had developed together previously that allows for the prediction of chemical shifts directly from the structure.
ShiftML uses DFT calculations for training, but can perform accurate predictions on new structures without performing additional quantum calculations. The method can calculate chemical shifts for structures with 100 atoms in seconds, which is a factor of as much as 10,000 compared to current DFT chemical shift calculations. The method does not depend on the size of the structure or the number of atoms. The stage for calculating chemical shifts is set by this.
The team used ShiftML to predict shifts on more than 200,000 compounds from the CSD, and then related the shifts to representations of the molecular environments. The graph that was constructed was a representation of the bonds between the atoms in the molecule. They were able to get statistical distributions of chemical shifts for each motif by bringing together all the identical instances of the graph. The representation is a simplification of the covalent bonds around the atom in a molecule and doesn't contain any 3D structural features.
After constructing the chemical shift database, the scientists looked to predict the assignments on a model system and applied the approach to a set of organic molecules for which the carbon chemical shift assignment has already been determined.
The framework was evaluated on a benchmark set of 100 crystal structures with between 10 and 20 different carbon atoms. They used the ShiftML predicted shifts for each atom as the correct assignment and excluded them from the statistical distributions used to assign the molecule. The two most probable assignments were found in more than 80% of the cases.
The method could speed up the study of materials by streamlining one of the first steps.
More information about the assignment of chemical shifts in organic solids is provided by the authors. Science.org has a DOI of/10.1126/sciadv.abk2341.
Science Advances and Nature Communications are journals.
The who's who problem in the NMR spectrum of organic crystals was solved by machine learning.
The document is copyrighted. Any fair dealing for the purpose of private study or research cannot be reproduced without written permission. The content is not intended to be used for anything other than information purposes.