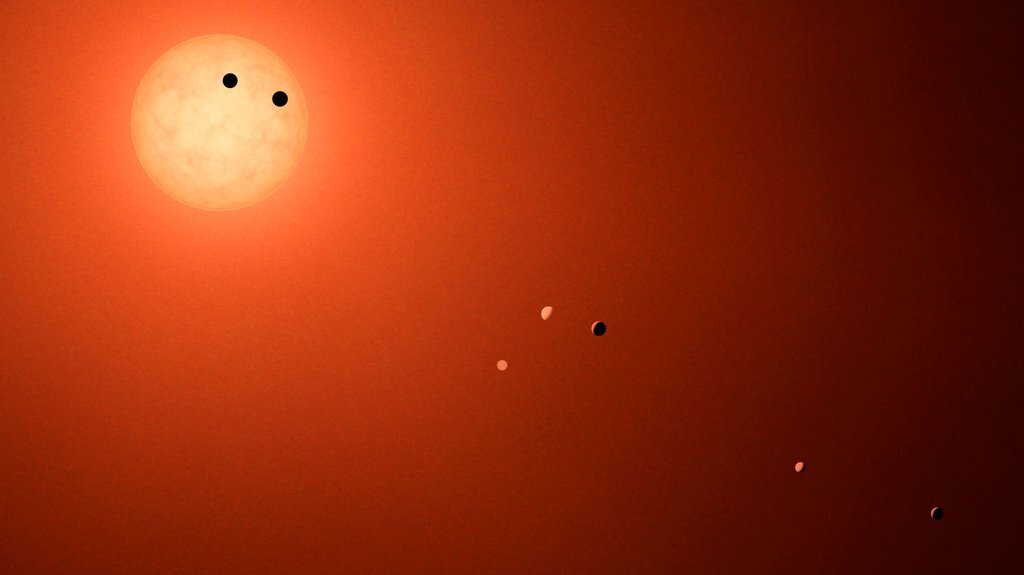
Scientists think our galaxy contains millions of planets, despite the fact that over 4,5000 planets have been found around other stars. There are many ways to detect small, faint bodies around bright stars. Credit: Caltech.
301 new exoplanets were recently added to the tally. The 4,569 already validated planets are now joined by the throng of planets. How did scientists discover so many planets at once? The answer is with a new deep neural network.
Machine learning methods using deep neural networks automatically learn a task when given enough data. NASA's Supercomputer, Pleiades, is the basis of the new deep neural network called ExoMiner, which can distinguish real exoplanets from imposters. Human experts use various tests and properties to confirm new exoplanets. Past confirmed exoplanets and false positive cases are used to learn.
People who are pros at combing through data and figuring out what is and isn't a planet can get supplements from ExoMiner. The data was gathered by NASA's K2 mission. It's a huge task to sift through a huge amount of data for a mission with thousands of stars in its field of view. This dilemma was solved by the ExoMiner.
"ExoMiner isn't a black box, there is no mystery as to why it decides something is a planet or not," said Jon Jenkins, exoplanet scientist at NASA's Ames Research Center in California's Silicon Valley. "We can easily explain the features of the data to reject or confirm a planet."
What is the difference between a confirmed exoplanet and a validation exoplanet? When different observation techniques reveal features that can only be explained by a planet, it's confirmed. Statistics are used to determine how likely or unlikely a planet is.
In a paper published in the Astrophysical Journal, the team at Ames shows how the 301 planets were discovered using data from the remaining set of possible planets. All 301 machine-validated planets were discovered by the Kepler Science Operations Center and then promoted to planet candidate status. No one was able to confirm them as planets until the invention of the ExoMiner.
When a planet crosses between us and its star, the star is dim because the planet is blocking out some light. Scientists use this method to find exoplanets. A light curve is a plot with a star and time. Scientists can see how much light the star blocks and how long it takes the planet to cross the disk of the star using this plot. The NASA's Goddard Space Flight Center is pictured.
The paper shows how ExoMiner is more precise and consistent in ruling out false positives and better able to reveal the genuine signatures of planets around their parent stars, all while giving scientists the ability to see in detail what led to its conclusion.
"When you hear something is a planet, you can be sure it's a planet," said Hamed Valizadegan, the machine learning manager with the Universities Space Research Association at Ames. "ExoMiner is more reliable and accurate than both existing machine and human experts because of the biases that come with human labeling."
None of the newly confirmed planets are thought to be Earth-like or in the right place to be in the right stars. They are similar to the population of confirmed exoplanets in our neighborhood.
"These 301 discoveries help us better understand planets and solar systems beyond our own, and what makes ours so unique," he said.
The European Space Agency's upcoming PLAnetary Transits and Oscillations of stars, or PLATO, mission, and NASA's Transiting Exoplanet Survey Satellite, or TESS, are just some of the missions that are being used to search for more exoplanets.
Valizadegan said that they can transfer the learning from the training of ExoMiner to other missions, including TESS, which they are currently working on. There's room to grow.
The Astrophysical Journal has information.
The new deep learning method adds 301 planets to the total count.
The document is copyrighted. Any fair dealing for the purpose of private study or research cannot be reproduced without written permission. The content is not intended to be used for anything other than information purposes.