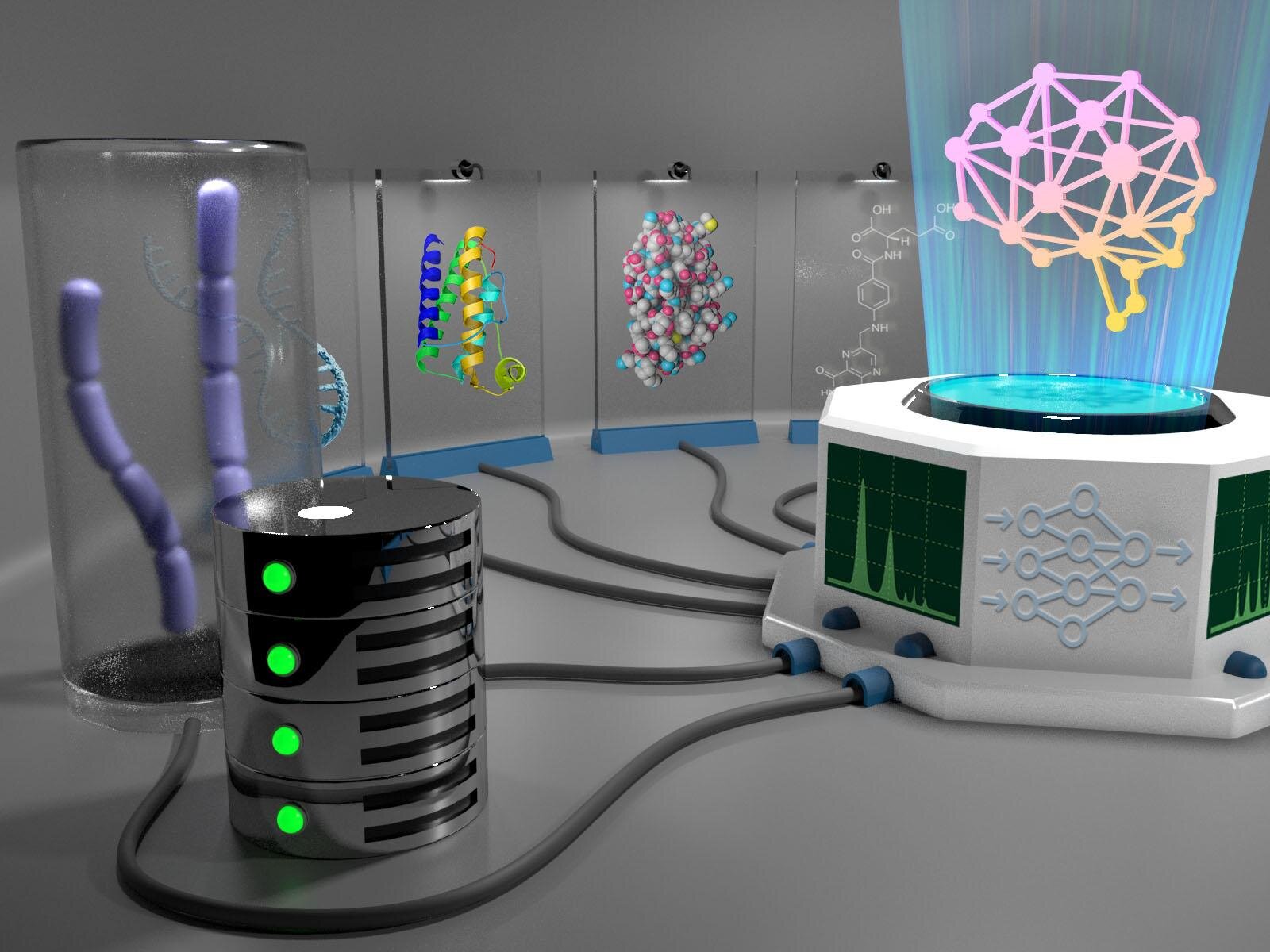
New yeast can be designed with machine learning and Bayesian inference. The Pacific Northwest National Laboratory has a credit.
Fossil fuels are harmful to the environment. It's hard for us to stop using them. Economic reasons are part of the answer. Fossil fuels are used in many parts of our society, from the energy grid to the manufacturing of certain textiles. It will come at a cost.
What if we could replace fossil fuels in an economically attractive way? A new research from the Pacific Northwest National Laboratory suggests a way to do that. Biologists have come up with a way to engineer yeast to produce itaconic acid using data integration and supercomputing power.
metabolic modeling is being used to create microbial factories.
Itaconic acid has a lot of potential. It could be used to substitute for fossil-fuel derived products. In 2004, it was named one of the top value added chemicals from biomass by the Department of Energy. Data scientist Neeraj Kumar saw the potential of itaconic acid as a replacement for petrochemicals.
Kumar and his colleagues had developed a way to calculate how engineered changes in microbes could affect metabolism. Kumar wanted to see if he could use these predictions to engineer yeast to produce high amounts of itaconic acid.
Kumar said that they needed to identify what genes in the itaconic acid production pathway they could alter so the yeast could make more of the chemical. The challenge was to find a balance between bioproduction and cellular health.
The Yarrowia lipolytica yeast is shown off by a Biologist. The Pacific Northwest National Laboratory has a credit card.
Design-build-test- learn.
Itaconic acid is produced by a few organisms. The Yarrowia lipolytica was given the ability to produce the chemical by the scientist. When Kumar approached her to collaborate, she was working on a modified yeast containing several different genes. The data from the metabolism and proteomic profile of the modified yeast was passed on to Kumar.
Kumar and his research associate Andrew McNaughton used machine learning to see what non essential genes could be removed from the yeast, or what helpful ones could be added, to increase the production of itaconic acid.
It was time to build after they selected the genes. Different versions of the yeast were created with genes added or removed based on Kumar and McNaughton's predictions. She tested the different yeasts to see if carbon flow was affected. Computational predictions from the data from the experiment matched the predictions from the future analysis.
It is exciting to see the potential of the research. "Machine learning and causality inference can uncover new ways of thinking about how a complex cell system, like yeast, could respond to individual gene changes, beyond what is possible from metabolic modeling alone."
The potential of metabolic modeling is expanded by machine learning and multiomics datasets.
Useful chemicals can be produced by yeasts and other microbes. It is easy to get them to produce some chemicals in high yields, but other chemicals may be more difficult. Kumar hopes that this system of combining machine learning with modeling will help overcome production challenges.
Kumar said there is an amazing potential to expand this model to other systems. "This strategy could open up a new era in bio system design for the production of eco-friendly chemicals."
Andrew D. McNaughton and his team have a paper on integrating Yarrowia lipolytica Multiomics with Metabolic Modeling. There is a book titled "acssynbio.1c00267."
The journal contains information about synthetic biology.
There is a news story about designing microbe factories for sustainable chemicals.
The document is copyrighted. Any fair dealing for the purpose of private study or research cannot be reproduced without written permission. The content is not intended to be used for anything other than information purposes.