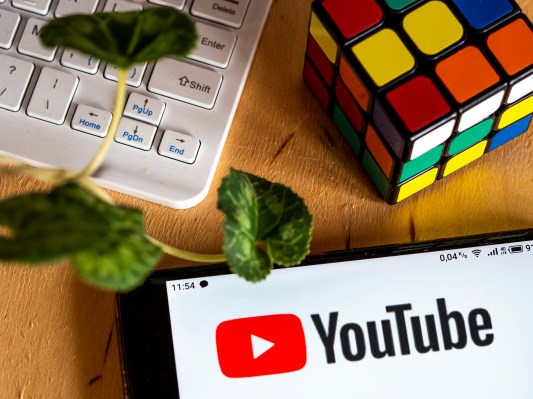
If the recent debates about the future media have had any impact on the English language, it has been in the form of disinformation, misinformation and infotainment. There has been much invective, fear and confusion about what social media is doing for us. This includes our individual psychologies, neurologies, and wider concerns about democratic societies. Joseph Bernstein recently stated that the shift from wisdom among the masses to disinformation was quite abrupt.
What is disinformation? Is it possible? If so, how can we find it and what are the best ways to identify it? Do we really care about the algorithms that our favorite platforms use to grab our attention? Noah Giansiracusa was attracted to the subject because of these complex mathematical and social science questions.
Professor at Bentley University in Boston, Giansiracusa is a mathematician who focuses his research on areas such as algebraic geometry. However, he has a tendency to look at social issues through a mathematical lens like connecting computational geometry with the Supreme Court. He recently published How Algorithms Prevent Fake news. This book explores some of the difficult questions surrounding the media landscape and the technology that is accelerating and ameliorating these trends.
Giansiracusa was my guest on a Twitter Space. Twitter doesn't make it easy to hear these talks afterward (ephemerality! I decided to pull the best bits from our conversation for posterity and you.
This interview has been edited for clarity.
Danny Crichton: What made you decide to write this book and research fake news?
Noah Giansiracusa : I noticed that there is a lot of interesting sociological and political science discussion about fake news and such things. On the technical side, Mark Zuckerberg will say that AI is going to solve all of these problems. It seemed difficult to bridge this gap.
You've probably all heard the Biden quote, "They are killing people" in relation to misinformation on social networks. These politicians are able to speak about things that they don't understand the algorithmic side of, but it is difficult for them. Computer science professionals are more interested in the details. I'm somewhere in the middle, so I don't really consider myself a hardcore computer scientist. It's easier to look at things from a different perspective and see the big picture.
At the end, I felt like I wanted to explore more interactions with society in places where things get messy and where math isn't so simple.
Crichton: Crichton comes from a mathematical background and is entering a contentious area that many people have written from different angles. What is the best approach and how can people miss some nuance in this area?
Giansiracusa - There is a lot to be proud of in journalism. I was amazed at the way that many journalists were able deal with technical issues. One thing I think they did right, but it was something that struck me: There are many times when an academic paper or an announcement from Google, Facebook, or any other tech company, and the journalist might mention it and extract a quote. But they seem afraid to actually look at it and try to understand it. It doesn't seem like they were able to. It seems more intimidating and fearful.
As a math teacher, one thing I have learned a lot is that people are afraid to say something wrong or make a mistake. This is especially true for journalists writing about technical topics. They don't want to make mistakes. It is easier to quote a Facebook press release or a professional expert.
Pure math is fun because you don't have to worry about making mistakes. You just try new ideas and see the results. You need to check all details before you start writing a paper or giving a presentation. Most of math is a creative process, where you are exploring and seeing how ideas interact. Although I thought my training as a mathematician would have made me more fearful about making mistakes and being very precise, it actually had the opposite effect.
Second, many of these algorithmic things are not as difficult as they appear. I'm not going to be sitting there programming them. Deep learning is the basis of many modern algorithms. It doesn't matter if you have a neural net. What architecture they use is irrelevant to me. All that matters is what the predictors are. What are the variables you feed this machine-learning algorithm? What is it trying output? These are simple concepts that everyone can grasp.
Crichton: The lack of transparency is one of the biggest challenges in analyzing these algorithms. Contrary to pure mathematics, which is a community made up of mathematicians working together to solve problems, many companies can be very antagonistic about providing data and analysis to the larger community.
Giansiracusa : There seems to be a limit on what one can infer from just being outside.
YouTube academic teams wanted to investigate whether YouTube's recommendation algorithm leads people down conspiracy theory rabbit holes of extremism. This recommendation algorithm uses deep learning. It is based upon your search history, demographics, and how many videos you have watched. You and your experience are so individualized that I was able find all of the studies incognito mode.
They are basically a user with no search history or information. They will go to a video, then click on the recommended video. Let's see where the algorithm takes us. This is a completely different experience from a human user with a past. This has been extremely difficult. I don't think anyone has found a way to algorithmically examine YouTube from the outside.
The only way I can see how you could do this is to do it in an old-school study. You would recruit volunteers, put a tracker on each person's computer, and then say, Hey, just do your normal life with your history and tell us about the videos you are watching. It is difficult to understand how to analyze that data in aggregate.
It's not me, or anyone else outside, who is having trouble because they don't have the data. Even people working in these companies know the algorithm on paper but don't know how it will behave in reality. It's like Frankenstein's monster: They built it, but don't know how it will work. It is only possible to really understand it if those with the data are willing to go the extra mile and invest their time and resources in it.
Crichton: There are many metrics that can be used to evaluate misinformation and determine engagement on a platform. Do you believe these measures are robust, based on your mathematical background?
Giansiracusa - People attempt to discredit misinformation. They might also comment on the misinformation, retweet or share it. This is considered engagement. These engagement metrics are often based on positive engagement. It all ends up getting lumped together.
This is also true for academic research. Research success is measured by how many citations it receives. It's not true that Wakefield's original autism paper and vaccines paper received tons of citations. Some were people citing them because they believed it was right. But many were scientists debunking the theory, who cite it in their paper to show that it is false. However, a reference is still a reference. It all contributes to the success metric.
That's what I believe is happening with engagement. How does the algorithm determine if I support it or not if I leave comments like, Hey, that's crazy! They could try some AI language processing, but I'm not sure if they are. It takes a lot of effort.
Crichton: Last but not least, I'd like to speak a little bit about GPT-3 as well as the concerns around fake news and synthetic media. Many fear that AI bots will overpower media with disinformation. How scared should we be?
Giansiracusa - My book grew from a class of experience. I wanted to be impartial and inform people so they could make their own decisions. I tried to get through the debate and let both sides talk. The newsfeed algorithms and recognition algorithms can amplify a lot of dangerous stuff and it is devastating for society. There are also amazing achievements in using algorithms to reduce fake news.
These techno-utopians claim that AI will fix everything. They have truth-telling, fact-checking, and algorithms that can detect misinformation, and then take it down. While there is some progress, it will not be enough. It will always depend on humans. The other thing is irrational fear. This hyperbolic AI dystopia is where algorithms are so powerful that it seems like they're going to take our lives.
Deep fakes first hit the news in 2018. GPT-3 had been released two years earlier. There was a lot fear back then. The main problem is psychological and economic.
So the original authors of GPT-3 have a research paper that introduces the algorithm, and one of the things they did was a test where they pasted some text in and expanded it to an article, and then they had some volunteers evaluate and guess which is the algorithmically-generated one and which article is the human-generated one. They were able to get very close to half the accuracy of their original paper, which is a mere 0.2% above random guesses. This sounds both incredible and frightening.
However, if you examine the details, it was like a single line headline to a paragraph. You'll start to notice the differences if you try to write a long article in The Atlantic or New Yorker. This was not something that the authors of this paper mentioned. They just did their experiment and thought, "Look at how successful it is!"
It looks so convincing that they can create these amazing articles. Here's the real reason why GPT-3 hasn't been as transformative in fake news, misinformation, and all that stuff. Fake news is mostly garbage. You could pay your nephew, 16 years old, to write a few fake news articles.
This is not because math made it easier for me to see. The main thing we are trying to do in mathematics, is to be skeptical. These things should be questioned and you need to be skeptical.